.png)
Imagine your computer understanding how you feel while you learn - that's what emotional analysis machine learning brings to education today. This AI technology reads your facial expressions, listens to your voice, and watches how you interact with learning materials, all to make your educational experience better. When you're frustrated, it makes lessons easier; when you're bored, it spices things up; and when you're excited, it keeps that momentum going. Teachers get helpful updates about how students are doing emotionally, letting them step in at just the right moment. It's like having a smart learning buddy that knows exactly when to change things up to keep you interested and learning at your best.
Key Takeaways
- Emotional analysis systems use real-time facial recognition and sentiment tracking to monitor student engagement and adjust learning content accordingly.
- AI-powered algorithms analyze emotional indicators to personalize learning paths and maintain optimal challenge levels for each student.
- Integration with VR and AR creates immersive educational experiences that adapt based on learners' emotional responses and comprehension.
- Teachers receive actionable insights from emotional analysis data to make timely interventions and support struggling students effectively.
- Longitudinal emotional pattern tracking helps predict learning barriers and enables proactive adjustments to educational content delivery.
Understanding Emotional Analysis in Machine Learning

The core components of emotional analysis systems rely on specialized software modules to process and interpret user emotional states through various data inputs.
You'll need to implement three primary elements: data collection interfaces that gather emotional indicators like facial expressions or text sentiment, processing algorithms that analyze these signals in real-time, and response mechanisms that adjust your learning content based on the emotional understandings.
Deep reinforcement learning techniques have shown a 20% improvement in cross-language emotion recognition compared to traditional supervised methods, particularly valuable for multilingual applications (Rajapakshe et al., 2022).
For ideal performance in your adaptive learning platform, consider integrating pre-trained machine learning models that can identify emotional patterns while maintaining user privacy and data security standards.
Why Trust Our AI Emotion Analysis Expertise?
At Fora Soft, we've been developing AI-powered multimedia solutions for over 19 years, with a particular focus on implementing artificial intelligence features across recognition, generation, and recommendation systems. Our experience in developing video surveillance and e-learning platforms has given us unique insights into emotional analysis implementation. We've successfully integrated AI recognition systems across numerous projects, maintaining a 100% project success rating on Upwork - a testament to our technical expertise in this field.
Our work with real-time video processing and AI recognition systems has been particularly valuable in developing emotional analysis solutions. Through our experience in telemedicine and e-learning platforms, we've refined our approach to implementing facial recognition, voice pattern analysis, and multimodal emotional input systems. Our team's expertise in WebRTC and various multimedia servers ensures robust, real-time emotional analysis capabilities that maintain high performance while protecting user privacy.
💡 Wondering how we implement these complex emotional analysis systems? Let's chat about your specific needs and explore solutions that match your vision. Our team has delivered successful AI projects for 19+ years.
Book a no-pressure discovery call - we'll share real examples from our portfolio.
Core Components of Emotional Analysis Systems
When building emotional analysis capabilities into your learning software, you'll need to implement three core technical components.
Your system should include computer vision algorithms for facial expression recognition that can detect subtle changes in users' expressions and map them to emotional states.
You can enhance the analysis by combining visual data with natural language processing to evaluate text sentiment and speech patterns while also integrating multiple data streams through a unified emotional scoring framework that processes inputs from various sensors and user interactions.
Facial Recognition and Computer Vision
Integrating facial recognition and computer vision capabilities into flexible learning systems offers considerable viewpoints into learners' emotional states during educational activities.
Real-time facial expression recognition systems can accurately classify emotions with over 90% accuracy in classroom environments, enabling immediate teacher responses to student emotions (Fakhar et al., 2022).
You can implement real-time emotion detection using computer vision libraries like OpenCV or TensorFlow, which analyze facial expressions during learning sessions. Enhanced facial emotion detection algorithms help track engagement levels and adjust content delivery based on detected emotional responses.
Natural Language Processing and Text Analysis
Natural Language Processing (NLP) and text analysis form three essential pillars of emotional analysis systems in adjustable learning environments. The application of NLP technology in online education has been shown to increase content analysis efficiency while revealing crucial learning patterns and trends (Niu, 2024).
You'll need to implement sentiment analysis APIs to process student comments, integrate emotion detection libraries to analyze text patterns, and develop machine learning models that track emotional changes through written responses. These components help you understand learners' emotional states more effectively, ultimately personalizing learning experiences and enhancing student engagement.
Our experience with Translinguist demonstrates the practical application of NLP in emotional analysis. By implementing advanced speech recognition and translation capabilities, we've created a system that not only translates words but also preserves emotional context across multiple languages.
Multimodal Emotional Input Integration
Beyond text analysis, multimodal emotional input systems combine different data streams to create a more complete picture of user emotional states.
By implementing multi-modal emotion recognition, you'll enhance your application's ability to process user reactions through various channels.
To effectively capture and analyze these diverse emotional cues, consider these key integration strategies:
- Integrate facial expression analysis using computer vision APIs
- Add voice tone detection through audio processing libraries
- Implement gesture recognition with motion tracking SDKs
Advanced Implementation Strategies
Your technical architecture for emotional analysis should integrate multiple data processing layers, including facial recognition APIs, voice pattern analysis, and behavioral tracking modules that work together seamlessly.
You'll need to implement real-time processing capabilities using distributed computing frameworks to handle the continuous stream of emotional data from your learning platform's users.
Consider incorporating flexible feedback loops that automatically adjust the system's response based on detected emotional states, allowing your platform to provide personalized learning experiences that evolve with each student's emotional journey.
Technical Architecture for Emotional Analysis
To build an effective emotional analysis system for your flexible learning platform, you'll need to choose between popular frameworks like TensorFlow or PyTorch for the machine learning components while integrating them with your existing tech stack.
Your data processing pipeline should incorporate real-time emotion detection through webcam feeds, voice analysis, or interaction patterns, with the processed data stored in scalable solutions like MongoDB or Amazon DynamoDB.
Consider implementing a microservices architecture that separates emotional analysis functions from the core learning platform, allowing for independent scaling and easier maintenance of each component.
The development of Translinguist required careful consideration of technical architecture to handle real-time translation and emotional analysis. Our implementation of three integrated services - speech-to-text, text-to-speech, and text-to-text - showcases how modular architecture can support complex emotional analysis requirements while maintaining system efficiency.
Framework Selection and Integration
Selecting the right framework for emotional analysis integration requires careful consideration of both technical capabilities and implementation requirements.
When implementing emotion detection models, you'll need to evaluate deep learning methods that align with your product's needs.
To make an informed decision and ensure a successful integration, prioritize these key framework attributes:
- Choose frameworks supporting real-time analysis
- Guarantee compatibility with existing data pipelines
- Verify scalability for growing user bases and increasing data volumes
Data Processing and Storage Solutions
Since emotional analysis generates substantial volumes of user interaction data, implementing strong data processing and storage solutions becomes essential for maintaining system performance. You'll need to select data processing frameworks that effectively handle emotional intelligence metrics while ensuring scalable storage solutions.
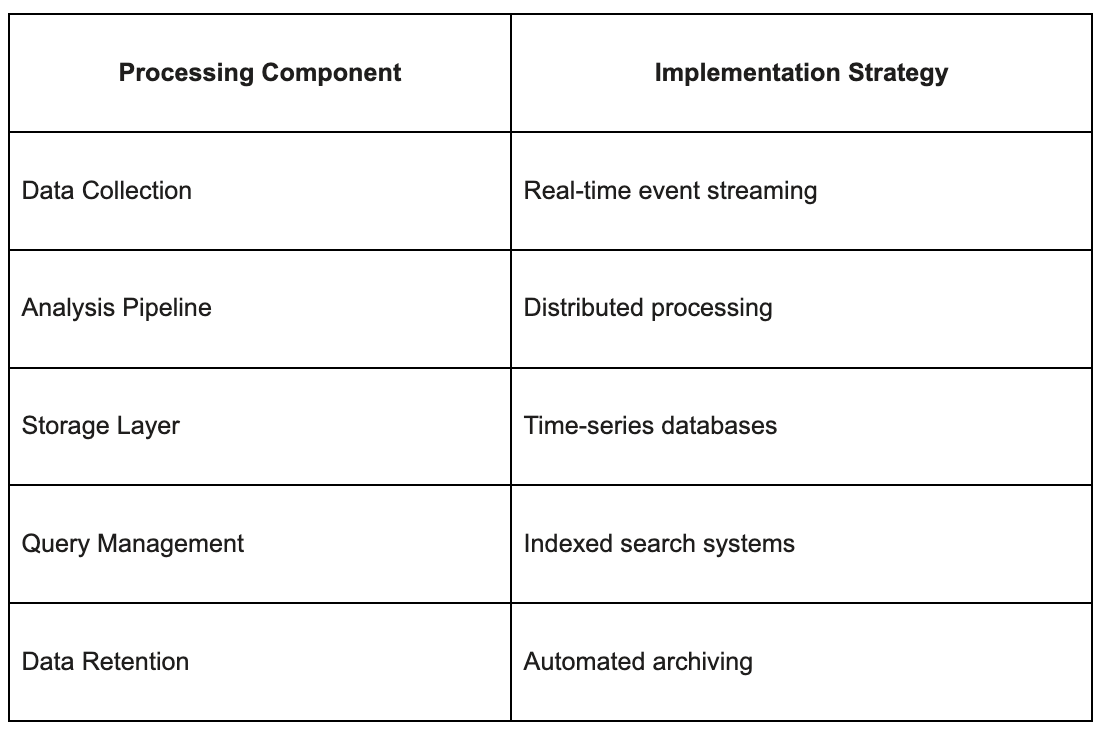
Real-time Emotion Detection Systems
To implement real-time emotion detection in your learning platform, you'll need to integrate various sensor inputs like webcams for facial recognition, microphones for voice analysis, and potentially biometric devices for physiological data.
Your system's processing pipeline should handle these multiple data streams simultaneously, using specialized APIs and SDKs to analyze emotional markers with minimal latency.
You can enhance the system's effectiveness by implementing a continuous feedback loop that captures user responses, validates emotional assessments, and automatically adjusts the detection parameters to improve accuracy over time.
Our work on Translinguist illustrates the practical application of real-time emotion detection in translation services. The platform analyzes voice patterns and speech characteristics to maintain emotional context during translation, ensuring that communication remains natural and effective across language barriers.
Sensor Integration and Processing
The successful integration of emotion detection sensors into versatile learning platforms requires careful consideration of both hardware compatibility and data processing pipelines.
When implementing emotion AI solutions, you'll need strong sensor integration protocols to guarantee reliable data collection.
To ensure robust and accurate emotion detection, focus on these critical aspects:
- Configure multi-sensor arrays to capture facial expressions, voice patterns, and biometric signals
- Implement real-time data preprocessing to filter noise and normalize sensor inputs
- Deploy synchronization mechanisms to align data streams from different sensor types
Feedback Loop Mechanisms
Implementing effective feedback loops stands at the core of real-time emotion detection systems, creating a dynamic bridge between user emotional states and flexible learning responses.
You'll need to develop automated feedback loop mechanisms that continuously monitor emotional experiences through API endpoints, triggering appropriate content adjustments based on detected patterns.
Consider implementing WebSocket connections for real-time data streaming and emotional state processing.
Enhancing Learning Through Emotional Intelligence

Online educators can leverage emotional intelligence tools to detect student engagement cues and adjust their teaching approach in real-time, creating more responsive and effective virtual learning environments
Your flexible learning platform's emotional intelligence capabilities can be enhanced by implementing real-time sentiment analysis modules that modify content delivery based on learner engagement signals.
You'll want to integrate teacher dashboards that display emotional analytics alongside performance metrics, enabling educators to make data-driven interventions through the AI system.
The platform should include configurable feedback loops where instructors can fine-tune how the AI responds to different emotional states, creating a more intricate and personalized learning experience.
Adaptive Content Delivery Systems
Your flexible learning system can employ real-time emotional analysis to create personalized learning paths that modify to each user's emotional state and comprehension level. Research shows that user satisfaction plays a crucial role in driving engagement and motivation within learning systems (Mohanty et al., 2022).
By monitoring indicators like completion time, error rates, and interaction patterns, you'll enable dynamic difficulty adjustments that prevent both frustration and boredom. This approach creates a positive feedback loop that enhances user interaction and sustained engagement with the learning material (Rafsanjani et al., 2022).
Implementing machine learning algorithms to analyze these metrics helps your system automatically calibrate content difficulty, ensuring users remain in an ideal learning zone while progressing through the material.
Personalized Learning Paths
While building flexible learning systems, integrating emotional intelligence into personalized learning paths creates a more responsive and effective educational experience.
Your deep learning model can analyze emotional responses to dynamically modify content delivery.
To effectively incorporate emotional intelligence into your personalized learning paths, consider these key strategies:
- Implement sentiment analysis to detect user frustration levels
- Configure adjustable difficulty scaling based on emotional states
- Design feedback loops that respond to engagement patterns
Dynamic Difficulty Adjustment
Integrating dynamic difficulty adjustment into flexible learning systems allows software to respond intelligently to a student's emotional state and performance metrics.
You can implement human emotion recognition through webcam analysis of facial expressions and track learning patterns to automatically calibrate content difficulty.
This personalization helps maintain ideal challenge levels, preventing both frustration from excessive difficulty and disengagement from overly simple material.
Teacher-AI Collaboration Framework
Your AI system can integrate real-time emotional analysis tools that monitor students' engagement levels and learning patterns through facial expressions, voice patterns, and interaction data.
You'll get actionable observations that help teachers identify when students are struggling, confused, or disengaged during lessons, allowing for timely interventions.
Real-time Student Insights
Through advanced machine learning algorithms, real-time student observations enable product owners to develop more responsive and personalized learning experiences.
Your software can monitor emotional expressions and learning patterns to modify content delivery instantly, with adaptive learning technologies showing improvements in educational outcomes by approximately 40% (Debeer et al., 2021).
To achieve this level of dynamic personalization, consider these key technological integrations:
- Implement facial recognition APIs to track student engagement levels
- Integrate sentiment analysis tools to assess text-based responses
- Deploy performance analytics modules to correlate emotional states with learning outcomes
Intervention Strategies
Effective intervention strategies in flexible learning systems require a balanced collaboration between AI algorithms and human instructors. You can implement emotion-detection features to gauge student engagement and modify content delivery automatically. Consider these proven approaches to enhance your responsive learning platform:

Real-World Implementation: Translinguist Platform
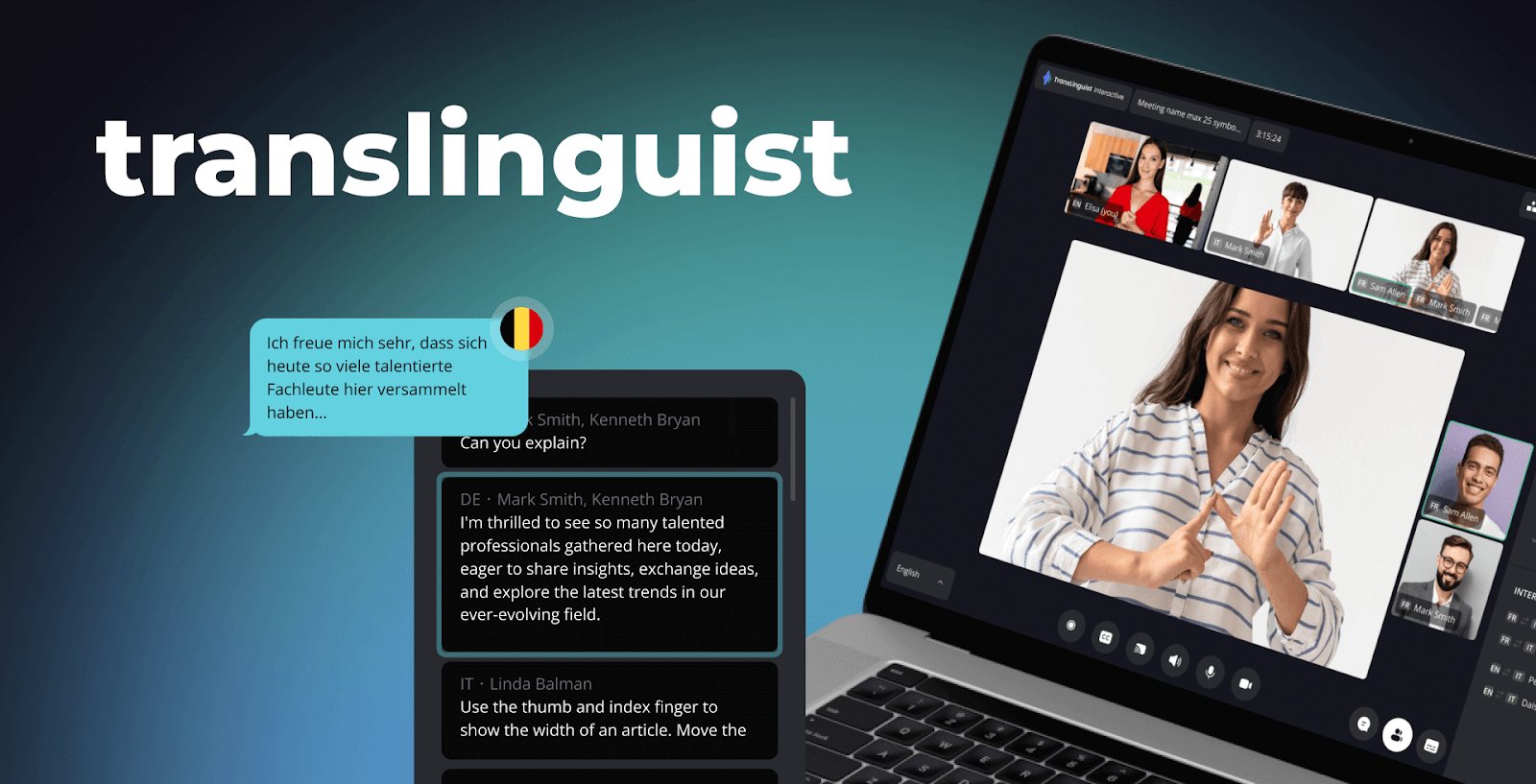
Through our development of Translinguist, we implemented advanced emotional analysis within our AI-powered interpretation platform. Our system processes real-time emotional indicators through facial expressions and voice patterns during video conferences to enhance translation accuracy. By integrating speech-to-text, text-to-speech, and text-to-text services, we created a seamless system that adapts to users' emotional states while delivering precise translations across 62 languages. The platform's ability to capture nuances like pace, intonation, and pauses demonstrates how emotional analysis can improve machine translation quality and create more natural communication experiences.
🌟 Inspired by Translinguist's success? Your project could be next. Our team is ready to bring emotional AI capabilities to your learning platform.
Check out our project portfolio or Start a conversation about your vision.
Ethical Considerations and Privacy
When implementing emotional analysis in your flexible learning software, you'll need strong data protection measures that safeguard user privacy and comply with regulations like GDPR and COPPA.
Your development team should integrate privacy-by-design principles, including data minimization, encryption, and clear user consent mechanisms for collecting emotional response data. These privacy measures not only ensure compliance but also significantly enhance user trust and engagement with digital platforms (Schmit et al., 2024).
To guarantee ethical AI development, you'll want to regularly test your algorithms for potential biases across different demographic groups and implement appropriate fairness metrics in your machine learning models.
Data Protection and User Rights
Implementing strong consent management in your flexible learning system lets you collect emotional analysis data while respecting user privacy through clear opt-in mechanisms and granular data-sharing controls.
You'll need to integrate privacy-preserving analytics that anonymize and aggregate emotional data before processing, using techniques like differential privacy and secure multi-party computation.
Consider developing a transparent data dashboard where users can view their emotional analysis metrics and modify their privacy settings, cultivating trust while maintaining compliance with data protection regulations.
Consent Management
The development of flexible learning systems requires a strong consent management framework to protect user privacy and guarantee ethical data handling.
When building your adjustable learning platform, consider these essential consent elements:
- Implement granular consent controls for collecting human emotion data.
- Provide transparent opt-out mechanisms that respect ethical considerations.
- Design clear data usage explanations in user-friendly interfaces.
Privacy-Preserving Analytics
Privacy lies at the heart of responsible emotion analytics implementation in flexible learning systems. You'll need to implement privacy-preserving analytics to protect user data while maintaining emotion detection capabilities. Consider these essential approaches:

Your system should process emotional data locally when possible, encrypt sensitive information, and minimize data storage duration.
Addressing Algorithmic Bias
When developing emotional analysis systems for flexible learning, you'll need to take into account how your algorithms respond to diverse cultural expressions of emotions and learning styles across different populations.
You can implement cross-cultural validation testing by gathering training data from multiple demographic groups and regularly auditing your system's performance across these segments.
To guarantee demographic fairness, integrate bias detection tools into your development pipeline and modify your models' parameters when they show statistically notable variations in accuracy between different user groups.
Cross-Cultural Considerations
Building flexible learning systems that work across different cultures requires careful consideration of algorithmic bias and cultural sensitivity.
You'll need to modify emotion labels and human-computer interaction patterns to match diverse cultural contexts.
To ensure your systems are culturally sensitive and effective, prioritize these key adaptations:
- Implement region-specific emotion recognition models that account for local expression norms
- Design culturally responsive user interfaces that respect local customs
- Configure feedback systems that align with cultural learning preferences
Demographic Fairness
How can flexible learning systems guarantee fairness across different demographic groups while processing emotional data? You'll need to implement regular bias testing and use a deep learning approach that validates emotional well-being metrics across diverse user groups. Consider incorporating these key fairness measures:
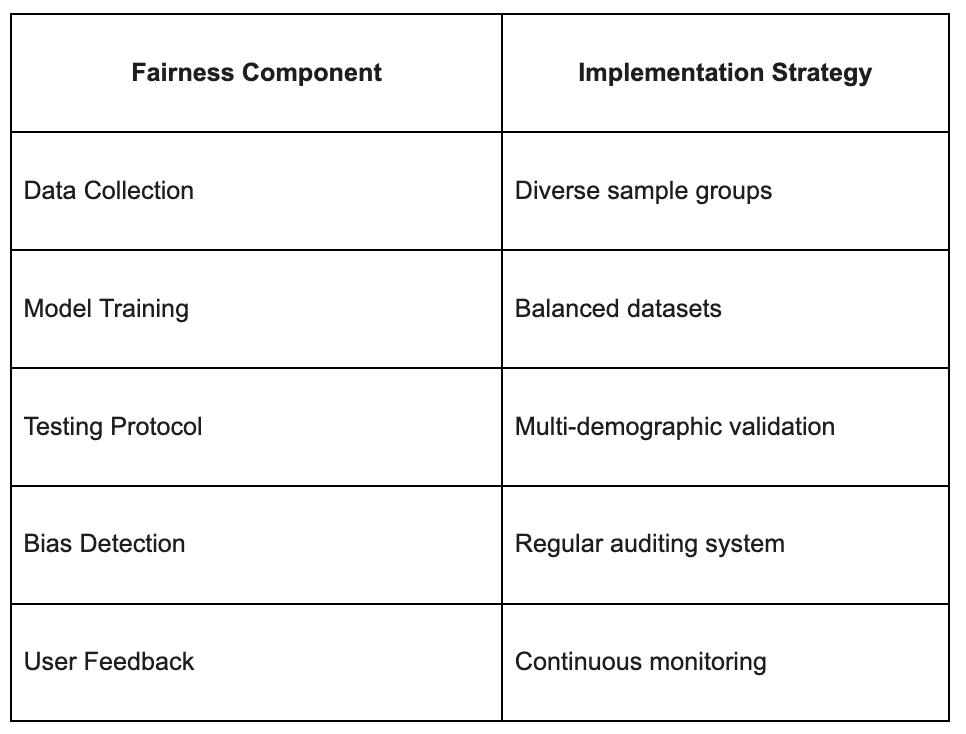
Future Developments and Trends
Extended reality technologies offer you robust new ways to integrate emotional analysis into your flexible learning platform, allowing for immersive experiences that can track facial expressions and body language in virtual environments.
Noteworthy opportunities in longitudinal analysis features can monitor emotional patterns across weeks or months of learning sessions, helping to identify trends in user engagement and emotional responses.
Extended Reality Integration
To enhance your adaptive learning platform's immersive capabilities, you'll want to integrate virtual reality (VR) and augmented reality (AR) components that respond to users' emotional states in real-time.
You can implement emotion-sensing algorithms that analyze facial expressions and biometric data through VR headsets or AR-enabled devices, allowing your system to modify the learning environment's difficulty, pacing, and presentation style.
Immersive Learning Experiences
Virtual and augmented reality technologies are revolutionizing flexible learning platforms by creating deeply immersive educational experiences. Studies show that when VR is integrated into educational environments, students demonstrate higher levels of motivation to engage with learning activities (Arents et al., 2021).
You can enhance your learning product by integrating emotion-aware features that respond to users' engagement levels.
To fully capitalize on these technologies and create truly engaging learning experiences, consider these emotion-aware and interactive features:
- Implement real-time facial expressions tracking to modify content difficulty
- Create emotion categories-based feedback loops for personalized learning paths
- Deploy interactive 3D simulations that adjust to learner responses
Emotion-Aware Virtual Environments
Building on the success of immersive learning features, emotion-aware virtual environments represent the next evolution in flexible educational technology.
You'll be able to integrate real-time analysis of emotions into your learning platforms through facial recognition APIs and biometric sensors.
This technology helps you modify content delivery based on learners' emotional states, creating more personalized and effective educational experiences.
Longitudinal Analysis Applications
You'll want to implement pattern recognition algorithms that track emotional states across extended periods, enabling your flexible learning system to identify recurring emotional barriers to learning.
These longitudinal patterns can feed into predictive learning models that anticipate when students might struggle, allowing your software to proactively adjust difficulty levels or introduce supplementary content.
Pattern Recognition
As development teams explore advanced pattern recognition algorithms, longitudinal analysis applications are becoming essential tools for tracking emotional patterns in versatile learning systems. To effectively leverage pattern recognition for enhanced emotional analysis, consider these critical implementation strategies:
- Implement real-time emotional analysis through facial recognition APIs to capture learner engagement metrics.
- Develop machine learning models that identify recurring behavioral patterns across learning sessions.
- Create flexible feedback loops that automatically adjust content difficulty based on emotional response patterns.
Predictive Learning Models
While pattern recognition focuses on current user behavior, predictive learning models employ historical data to anticipate future emotional states and learning needs.
Implementing these machine learning approaches can help your software forecast user struggles and automatically modify difficulty levels.
Frequently Asked Questions
How Can We Validate the Accuracy of Emotional Analysis Models?
You can validate emotional analysis models through A/B testing, user feedback surveys, cross-validation with expert-labeled data, continuous monitoring of model predictions, and comparison against established benchmarks for sentiment accuracy metrics.
What Programming Frameworks Are Best Suited for Emotional Analysis Implementation?
TensorFlow and PyTorch are best for emotion detection, while NLTK and spaCy work great for sentiment analysis. For real-time processing, consider using Fast.ai or Keras with pre-trained models.
How Do We Handle Multi-Language Support in Emotional Analysis Systems?
You'll need to implement language-specific NLP libraries, use Unicode support for text processing, and maintain separate sentiment dictionaries. Consider using frameworks like NLTK or spaCy with multilingual models for each language.
What Are the Hardware Requirements for Real-Time Emotional Processing?
You'll need a modern CPU (i5/Ryzen 5 or better), 8GB+ RAM, and a dedicated GPU for real-time emotion processing. Consider cloud processing solutions if you can't meet local hardware requirements.
Can Emotional Analysis Algorithms Be Integrated With Existing Learning Management Systems?
You can integrate emotional analysis APIs into your LMS through REST endpoints, WebSocket connections, or SDKs. Most modern systems support these integrations using JSON data exchange and webhook notifications.
To sum up
You're at the forefront of a transformative shift in educational technology as emotional analysis reshapes flexible learning. By implementing these advanced tracking systems and responsive algorithms, you'll create more engaging and personalized learning experiences. While privacy concerns require careful consideration, the potential benefits of emotion-aware education are substantial. Moving forward, you'll see this technology become an essential component of effective digital learning platforms.
🚀 Ready to transform your learning platform with emotional AI? Don't let this opportunity slip away. Our expertise in AI integration and proven track record make us the ideal partner for your project.
Schedule your free consultation today and let's discuss how we can bring your vision to life.
Comments