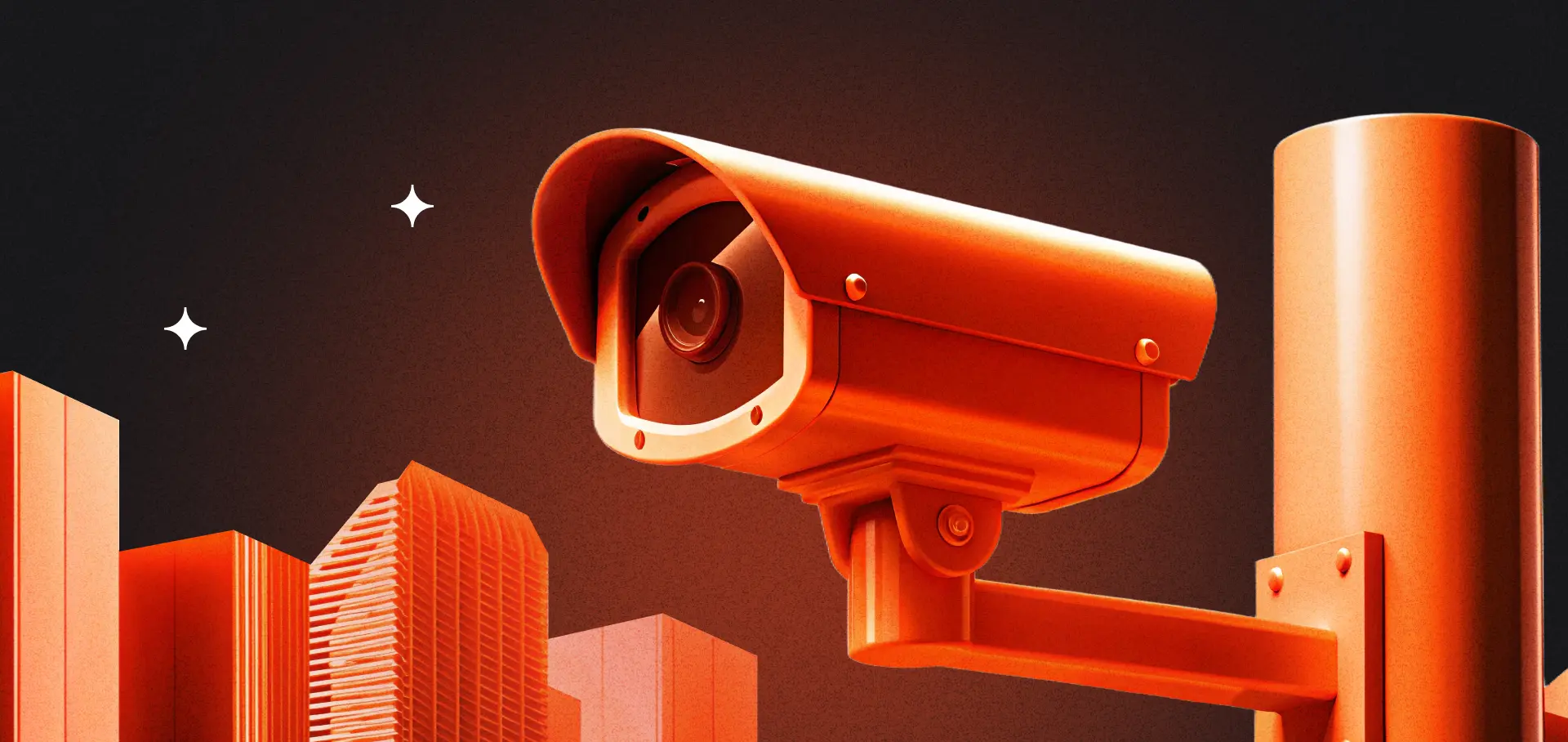
Modern video security has come a long way from simple recording systems. AI video analytics has changed how cameras work - they don't just record anymore, they understand what they're seeing. Think of it as giving your security cameras a brain that can spot objects, recognize faces, and even tell when something unusual is happening, all in real-time. The smart combination of local processing for quick responses and cloud computing for bigger tasks makes these systems both fast and powerful. What's really cool is how these systems keep data private through smart local processing, while still letting you choose between ready-made or custom AI solutions based on what you need. This mix of smarts and security is making video surveillance more helpful and reliable than ever before.
Key Takeaways
- AI enhances video security software through real-time video analytics.
- AI combines ML and CV for object detection, motion analysis, and behavior recognition.
- AI architecture improves efficiency with edge processing and cloud collaboration.
- AI models in video security anticipate threats with predictive analytics and anomaly detection.
- Privacy and transparency are enhanced through federated learning and explainable AI.
Understanding Modern AI Video Analytics

Surveillance technology has evolved from traditional cameras to AI-powered systems.
Modern AI video analytics uses machine learning and computer vision to enhance security. Core components include object detection, facial recognition, and behavior analysis, which help identify and track potential threats in real-time.
The integration of AI and machine learning in surveillance systems has demonstrated a 40% improvement in anomaly detection accuracy compared to conventional methods (Biu et al., 2024).
Why Trust Our AI Video Analytics Expertise?
At Fora Soft, we've been at the forefront of video surveillance and AI-powered multimedia solutions since 2005. With over 19 years of experience developing sophisticated video streaming software, we've successfully implemented AI recognition features across hundreds of projects, maintaining a 100% project success rating on Upwork. Our team has worked with over 450 client organizations, including police departments and medical institutions, giving us unique insights into the practical applications and challenges of AI video analytics.
Our expertise isn't just theoretical - we've hands-on experience implementing cutting-edge technologies like WebRTC, LiveKit, and Kurento, which are crucial for modern AI-powered video surveillance systems. We understand the intricate balance between sophisticated AI features and user-friendly interfaces, having developed solutions across multiple platforms including web, mobile, and smart TV applications. This comprehensive experience allows us to provide insights that bridge the gap between technical capabilities and practical implementation.
🎯 Ready to leverage AI video analytics expertise that police departments trust? Let's discuss your project!
→ Schedule a free consultation
→ See our successful implementations
Evolution from Traditional to AI-Powered Surveillance
The shift from traditional CCTV systems to AI-powered surveillance has been driven by the need for smarter, more efficient video analytics.
Traditional systems mainly record footage for later review, but they can't analyze or act on it immediately. The introduction of AI in camera systems has revolutionized this limitation by enabling real-time processing capabilities, effectively moving beyond traditional systems that only provide footage for later review (Nikita et al., 2023).
With artificial intelligence, cameras can now process and understand what they're seeing in real-time. This is called real-time video analytics. It's like giving the camera a brain that can spot unusual activities, identify faces, or even count people.
This evolution means that cameras aren't just passively recording anymore; they're actively helping to make spaces safer and more efficient. For product owners, this shift opens up new possibilities for features that could make their security systems more useful to end users.
Core Components and Technologies
Modern AI video analytics blend Machine Learning (ML) and Computer Vision (CV) to identify patterns and objects in real-time footage.
This integration is bolstered by an Edge-Cloud Hybrid Processing Architecture, where initial data processing occurs on local devices ( Edge ), and more intensive tasks are handled in the cloud.
This setup balances speed and efficiency, enhancing the system's overall responsiveness and accuracy.
Machine Learning and Computer Vision Integration
Integrating machine learning with computer vision is what's driving the latest advancements in AI video analytics. This combination allows software to identify patterns, detect anomalies, and make decisions based on visual data.
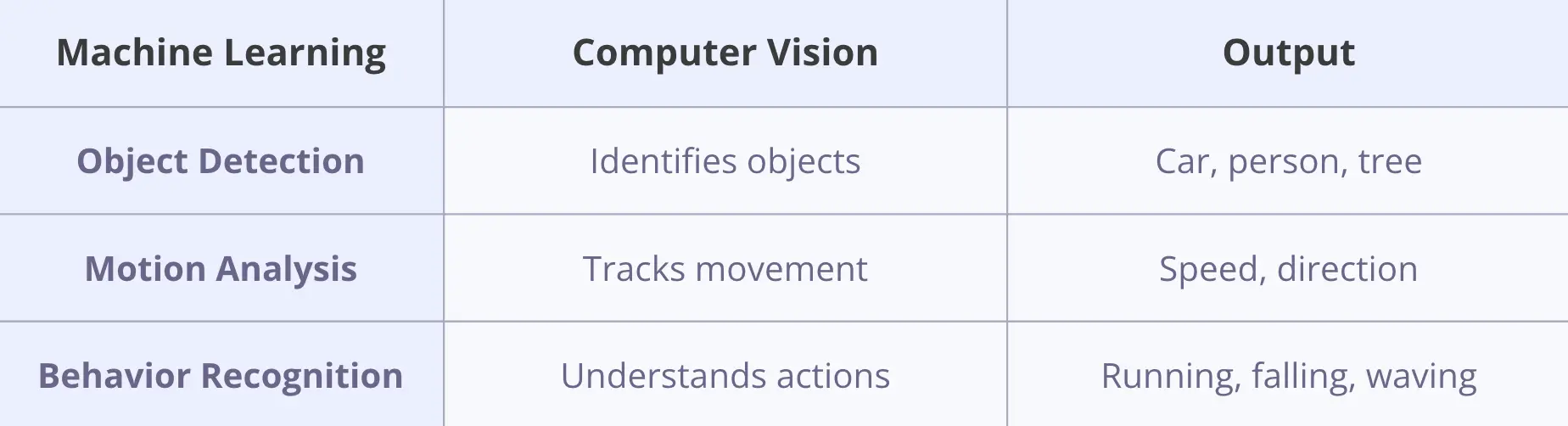
With these capabilities, video security systems can now understand what's happening in a scene, not just record it. This makes them more useful for end users needing quick findings. For instance, a system might alert users to unusual activities, like a car moving erratically. It's all about making video data more meaningful and easier to digest. This improvement is thanks to AI's ability to learn and interpret visual info just like a human would.
Edge-Cloud Hybrid Processing Architecture
Today, AI video analytics is becoming more efficient thanks to a setup called edge-cloud hybrid processing architecture.
This system splits tasks between local devices (edge) and remote servers (cloud).
The following are the key aspects:
- Reduced Latency: AI processing at the edge means quicker responses, vital for real-time applications.
- Bandwidth Efficiency: Less data needs to be sent to the cloud, reducing network traffic and cost.
- Scalability: The cloud handles heavy processing, so the edge devices stay light and efficient.
- Reliability: If the cloud goes offline, edge devices can still run basic AI-powered video analytics.
Real-World Implementation: V.A.L.T - Advanced Video Analytics in Action
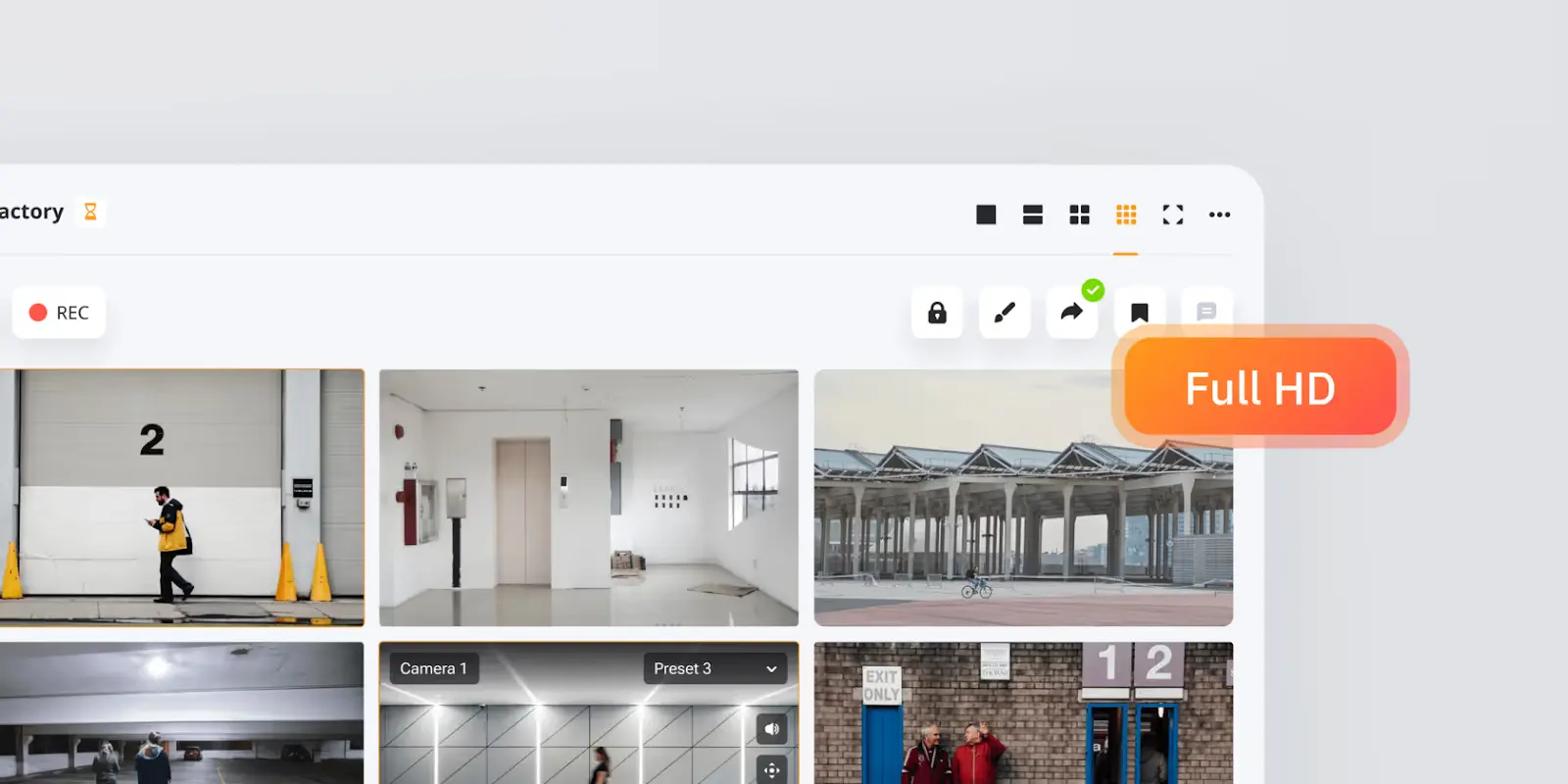
In developing V.A.L.T, our video surveillance system, we implemented cutting-edge AI analytics while prioritizing user accessibility. Our experience with over 450 client organizations, including police departments and medical institutions, taught us that successful AI integration requires balancing sophisticated features with intuitive usability.
The system demonstrates how edge-cloud processing can be practically implemented, featuring HD video streaming from multiple IP cameras with perfect audio-video synchronization, while maintaining high security through SSL and RTMPS encryption.
Transformative Features and Capabilities
AI in video security introduces robust features like real-time threat detection and response.
This means cameras can instantly spot and react to suspicious activities, alerting security teams right away.
Moreover, advanced privacy and security measures are built, insuring that sensitive data is protected while keeping an eye on potential threats.
Real-Time Threat Detection and Response
AI-powered video security systems are getting smarter with real-time threat detection.
These systems can now integrate different types of data video, audio, and even thermal imaging to spot and confirm threats.
With predictive analytics, the software doesn't just react to incidents but can also foresee and help prevent them.
Multimodal AI Integration (Video, Audio, Thermal)
Integrating multiple data sources like video, audio, and thermal imaging can considerably enhance the capabilities of video security systems.
This fusion, known as multimodal AI integration, enables the detection of potential threats in real time. Key features include:
- Improved Accuracy: Combining different data types reduces false alarms.
- Night Vision: Thermal imaging allows for 24/7 monitoring, even in low-light conditions.
- Audio Anomalies: Sound patterns can alert to unseen events, like breaking glass.
- Contextual Awareness: Understanding scene context enhances threat detection, such as recognizing unusual behaviors.
This integration allows systems to identify and respond to threats more effectively.
Predictive Analytics and Incident Prevention
Building on the enhanced detection capabilities of multimodal AI integration, predictive analytics takes video security a step further. By utilizing historical data and machine learning, security systems can now identify patterns that indicate potential risks. This means the system can perform real-time detection of anomalies, enabling faster threat detection and response. Imagine a system that doesn't just react to incidents but anticipates them, alerting authorities before an issue escalates.
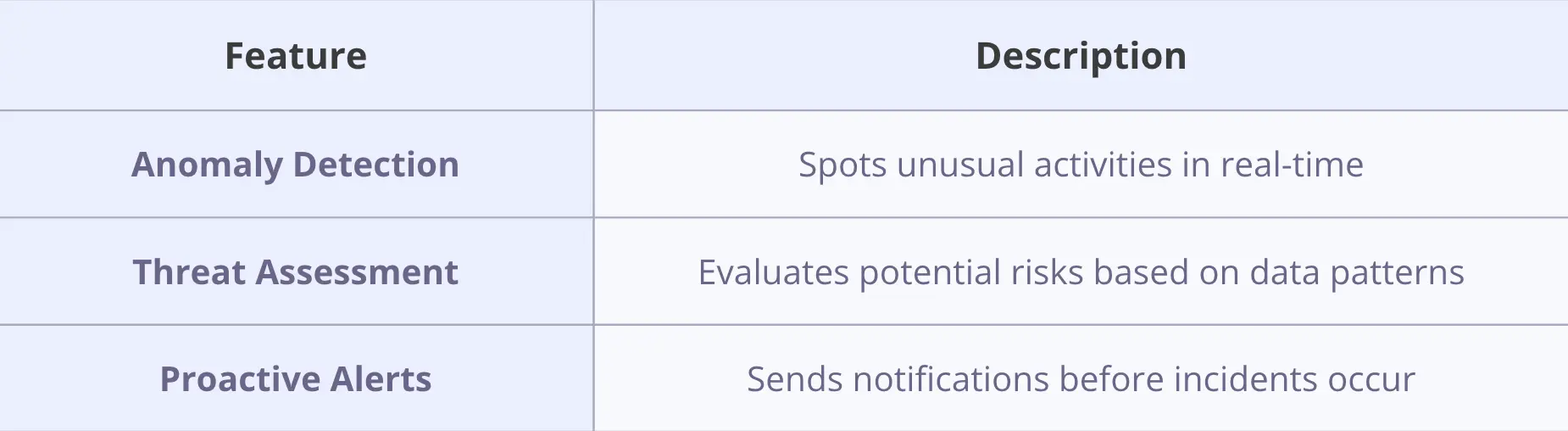
With predictive analytics, the system can analyze vast amounts of data quickly, making it easier to spot potential problems. This advancement helps in transforming traditional surveillance into proactive security measures, benefiting end users considerably.
Advanced Privacy and Security Measures
When it comes to enhancing AI in video security, developers are focusing on two key areas: federated learning and explainable AI.
Federated learning is a technique where the AI model is trained across many decentralized devices without exchanging the data itself, ensuring user privacy.
Furthermore, explainable AI is being used to make sure the system's decisions are clear and understandable, which is important for compliance with regulations.
Federated Learning Implementation
Although federated learning is not brand new, it's quickly becoming a breakthrough for video security. It allows AI-based video analytics to learn from multiple devices without sharing sensitive data.
This means better privacy and improved machine learning capabilities. Key advantages that make federated learning a game-changer in video security include:
- Local Data Processing: Data stays on the device, ensuring privacy.
- Collaborative Learning: Devices work together to improve models.
- Enhanced Security: No raw data is transferred, reducing risk.
- Scalability: Easily add more devices to the learning network.
This approach lets video security systems adjust quickly to new threats and patterns.
Explainable AI for Compliance
Incorporating explainable AI for compliance is revolutionizing video security, making it easier for users to understand why the system makes particular decisions. This transparency helps guarantee the AI complies with government regulations.
By providing a clear understanding of the AI's decision-making process, users can verify detection accuracy and maintain trust. For instance, in identifying unusual activities, explainable AI can highlight the specific behaviors that triggered an alert, enhancing overall system reliability and user confidence.
For product owners, this means adding a layer of accountability that's essential for sensitive applications like video surveillance.
Implementation Strategy and Future Outlook

To bring AI into video security, choosing the right AI architecture is key.
Next-generation integration approaches are combining AI with existing security systems. These approaches can make video security smarter and faster for end users.
Choosing the Right AI Architecture
When picking AI for video security, folks talk about whether to build something from scratch or use models already available, each having its pros and cons.
Custom models can be tailored to specific needs but require more resources, while pre-trained models are quick to set up but might not always fit perfectly. Recent research indicates that while custom models demand substantial resources, they offer greater organizational flexibility and can lead to improved customer satisfaction (Saad et al., 2023). In the context of image processing tasks, pre-trained convolutional neural networks have shown significant improvements in detection accuracy (Nfor et al., 2024).
Designing these systems to be sustainable and energy-efficient is vital, ensuring they work well without wasting energy or harming the environment.
Custom vs Pre-trained Models
Implementing AI in video security involves deciding between custom and pre-trained models, each with its own strengths and trade-offs.
In deep neural networks, developers can opt for a custom solution. This approach is flexible and can be tailored to very specific needs. However, it demands a lot of data and more time to train.
Here’s a breakdown of the choices:
Custom Models:
- Flexibility to meet specific needs.
- Requires lots of data for training.
- Longer development time.
- Can address unique security challenges.
Pre-trained Models:
- Quick to implement.
- Usually performs well on common tasks.
- May not fit specialized requirements.
- Easier to upgrade and maintain.
Sustainable and Energy-Efficient Design
In developing AI for video security, the choice of AI architecture greatly impacts the system's energy consumption and sustainability. Different artificial intelligence models require varying amounts of computational capability.
For instance, lightweight video analytics algorithms can run efficiently on edge devices, reducing the need for constant cloud processing. This not only saves energy but also guarantees quicker response times, as data doesn't have to travel to distant servers.
Meanwhile, more complex algorithms may demand high-performance hardware, consuming more energy. Future trends point towards optimizing AI architectures to balance performance and energy efficiency, benefiting both end-users and the environment.
Next-Generation Integration Approaches
Advancements in AI for video security are introducing platforms that don't need coding, making it easier to build solutions.
These no-code development platforms allow for automated workflow orchestration, which means tasks can be managed and sequenced automatically.
This leads to smoother integration and more efficient operations, improving the overall user experience.
No-Code Development Platforms
Developers often face the challenge of creating complex software without needing to write extensive code. That's where no-code development platforms come in.
They help developers build software using drag-and-drop interfaces instead of writing code line by line. These platforms are especially useful for integrating AI-enabled video analytics with real time processing.
Here are some key features:
- Easy Integration: Connect different tools and services without writing complex code.
- Quick Deployment: Get applications up and running faster than traditional coding methods.
- User-Friendly Interface: Simple drag-and-drop features make it accessible for non-technical users.
- Customization: Tailor applications to meet specific needs without deep programming knowledge.
These platforms are becoming popular among product owners looking to enhance their offerings. By using no-code platforms, developers can focus more on improving user experience and less on the technical complexities of coding.
Automated Workflow Orchestration
The simplicity and efficiency of no-code development platforms have paved the way for another essential trend in video security: automated workflow orchestration. This involves creating rule-based algorithms that automate tasks like sending alerts for lane departure.
Interactive AI Video Security Implementation Roadmap
Curious about implementing AI in your video security system? This interactive roadmap helps you visualize the journey from traditional surveillance to a fully AI-powered security solution. Explore each stage to understand the benefits, challenges, and key technologies involved. Based on our extensive experience in developing AI-powered multimedia solutions, this tool will help you grasp the progressive steps needed for successful implementation.
Frequently Asked Questions
Is AI Video Security Software Expensive?
The cost of AI video security software varies greatly. High-end solutions with advanced features can be expensive, while basic systems may be more affordable. Pricing depends on factors such as the level of AI integration, scalability, and customization requirements. Some providers offer flexible plans to accommodate different budgets.
Can AI Replace Human Security Guards?
While AI can enhance surveillance through pattern recognition and anomaly detection, replacing human security guards entirely is unlikely. AI lacks human judgment, intuition, and the ability to physically intervene or de-escalate situations. Instead, AI is best used to augment human capabilities.
What Happens if AI Misidentifies a Threat?
Mistaken identity by AI can lead to false alarms or overlooked threats. This inaccuracy may result in unwarranted actions, such as dispatching emergency services when not needed, or neglecting actual security breaches, potentially causing harm or loss. Regular AI audits and continuous learning updates are recommended to mitigate such risks effectively.
Will AI Invade Privacy in Video Surveillance?
AI in video surveillance may invade privacy by continuously monitoring individuals without clear consent or oversight. Misuse or data breaches can expose sensitive personal information. To mitigate, strict regulations and ethical guidelines should be enforced to protect civil liberties.
How Does AI Handle False Positives/Negatives?
AI handles false positives/negatives by applying techniques such as anomaly detection, probability thresholding, and continuous learning through feedback. It leverages algorithms that improve over time, refining their ability to distinguish between genuine threats and benign events. Cross-verification methods and ensemble models are also implemented to enhance accuracy.
To Sum Up
AI's role in video security software is transforming the industry. With advanced analytics like object detection and behavior recognition, these systems can quickly spot unusual activities and alert users in real-time. This makes security much faster and more reliable than traditional methods. Developers have options like cloud-based or edge computing architectures to make these systems even smarter and more efficient. Integrating AI with existing surveillance setups not only enhances threat detection but also guarantees better privacy and security measures. The future looks promising with continuous improvements and new features being added regularly. Over 6,000 developed nations have seen a sharp decline in security incidents post the introduction of AI surveillance tools as part of national security measures.
🎯 Ready to future-proof your video security with AI? Our team is here to help!
References
Biu, P., Nwasike, C., Tula, O., et al. (2024). A review of GIS applications in public health surveillance. World Journal of Advanced Research and Reviews, 21(1), 030-039. https://doi.org/10.30574/wjarr.2024.21.1.2684
Nfor, K., Armand, T., & Kim, H. (2024). A holistic approach to image forensics: Integrating image metadata analysis and ELA with CNN and MLP for image forgery. https://doi.org/10.21203/rs.3.rs-4667372/v1
Nikita, M., Kashyap, L., Singh, B., & Tyagi, D. (2023). AI enabled advance surveillance system. International Research Journal of Modernization in Engineering Technology and Science. https://doi.org/10.56726/irjmets37500
Saad, N., Elgazzar, S., & Kač, S. (2023). Investigating the impact of supply chain management practices on customer satisfaction through flexibility and technology adoption: Empirical evidence. Business Strategy & Development, 7(1). https://doi.org/10.1002/bsd2.326
Comments